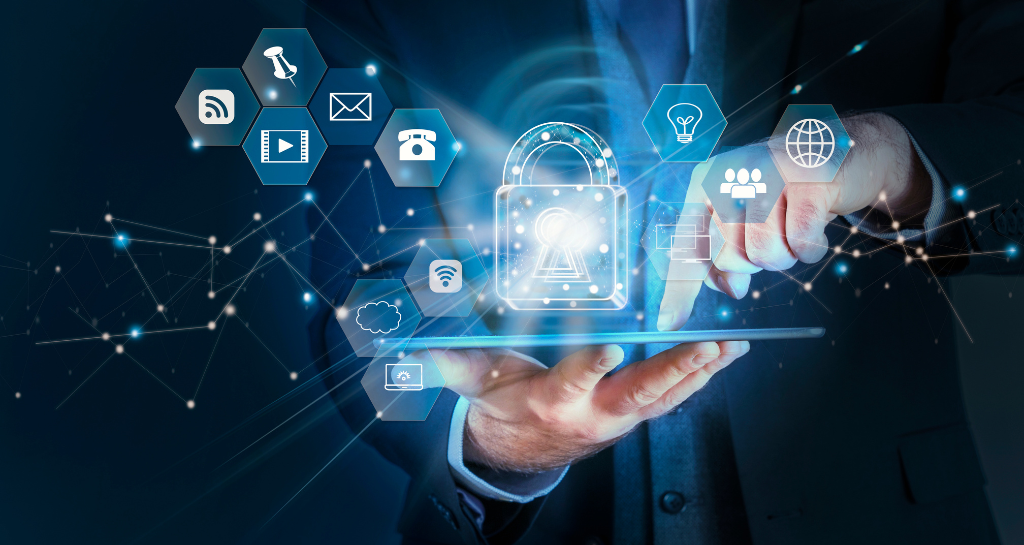
AI in Financial Services: Risk Management and Fraud Detection
Artificial Intelligence (AI) is rapidly transforming the financial services industry, offering sophisticated tools to enhance risk management and detect fraud. In an era where financial institutions handle massive volumes of transactions and sensitive data, the ability to quickly and accurately identify risks is essential. AI-driven solutions are providing financial firms with new ways to mitigate risks, improve compliance, and detect fraudulent activities in real time. As a technology consultant specializing in AI for financial services, I’ve seen the impact that AI can have on making risk management and fraud detection more efficient and effective. In this blog, we’ll explore how AI is revolutionizing these areas and what businesses need to know to leverage its power.
The Role of AI in Financial Risk Management
Financial risk management involves identifying, assessing, and mitigating risks that can affect a financial institution's profitability and reputation. These risks include credit risk, market risk, operational risk, and compliance risk. AI provides tools for automating the analysis of these risks by processing large volumes of data, identifying patterns, and predicting potential risks.
1. Predictive Analytics for Risk Assessment
AI uses predictive analytics to analyze historical data and identify trends that signal potential risks. Machine learning algorithms can learn from past events to predict future outcomes, allowing financial institutions to make informed decisions about credit, investment, and operational risks.
Example:
- Credit Risk Assessment: AI-powered systems can analyze vast datasets, including credit scores, transaction history, and behavioral data, to assess the likelihood of default for loan applicants. This helps lenders make faster and more accurate decisions about extending credit.
Benefits:
- Improved Decision-Making: AI provides actionable insights, helping businesses make more informed risk management decisions.
- Early Detection: AI identifies risks early, giving financial institutions more time to take corrective action.
2. Market Risk Management
AI systems can analyze real-time market data, financial news, and geopolitical events to assess the potential impact on a financial institution’s portfolio. These tools help traders and analysts manage exposure to market risks by providing timely alerts and recommendations.
Example:
- Automated Trading Systems: AI algorithms can identify market trends and execute trades automatically, mitigating risks associated with market volatility and reducing human error in decision-making.
Benefits:
- Real-Time Insights: AI provides up-to-the-minute analysis, allowing financial institutions to adjust their strategies in response to market fluctuations.
- Risk Reduction: By using AI to manage market risk, institutions can reduce their exposure to sudden changes in market conditions.
3. Operational Risk Management
AI can help identify operational risks such as system failures, process inefficiencies, or cybersecurity threats by analyzing internal data and external threats. AI-powered tools can monitor and flag unusual patterns that may indicate operational risks, enabling institutions to take proactive measures.
Example:
- Cybersecurity Risk Monitoring: AI tools can detect potential cybersecurity threats by continuously monitoring network activity for anomalies. This helps institutions prevent data breaches and protect customer information.
Benefits:
- Proactive Risk Management: AI tools can detect risks before they escalate, reducing operational disruptions.
- Improved Compliance: AI helps ensure that institutions comply with regulatory requirements by identifying potential compliance issues early.
AI in Fraud Detection for Financial Services
Fraud detection is one of the most critical applications of AI in financial services. AI systems use machine learning and pattern recognition to detect anomalies in transactions, alerting institutions to potential fraud before significant damage is done.
1. Real-Time Transaction Monitoring
AI-powered fraud detection systems monitor transactions in real time, flagging suspicious activities based on behavioral patterns, transaction history, and risk scores. These systems can learn from past fraudulent activities to better detect emerging threats.
Example:
- Credit Card Fraud Detection: AI can detect unusual spending patterns, such as a sudden large purchase or transactions in an unexpected location, and automatically flag or block the transaction.
Benefits:
- Speed and Accuracy: AI detects fraudulent activities in real time, reducing the window for potential losses.
- Reduced False Positives: AI can differentiate between normal and abnormal activity more effectively, minimizing false positives and improving the customer experience.
2. Anomaly Detection
AI uses anomaly detection algorithms to identify deviations from normal patterns. By analyzing large volumes of transaction data, AI can pinpoint irregularities that may indicate fraudulent activity, even if the fraudulent behavior has never been seen before.
Example:
- Suspicious Account Activity: AI systems can monitor account behavior for unusual activity, such as multiple login attempts from different locations or changes in spending habits, which may indicate account takeover fraud.
Benefits:
- Early Fraud Detection: AI can detect emerging fraud tactics before they become widespread, improving fraud prevention.
- Scalability: AI systems can analyze vast amounts of data quickly, making it easier for financial institutions to scale their fraud detection efforts.
3. Natural Language Processing for Fraud Detection
Natural Language Processing (NLP), a branch of AI, can be used to analyze unstructured data such as emails, text messages, or social media posts to detect potential fraud. NLP can identify suspicious communication patterns and flag high-risk interactions.
Example:
- Fraudulent Loan Applications: NLP can analyze written documents or social media posts related to loan applications to detect signs of fraud, such as inconsistencies in the applicant’s history or evidence of fake information.
Benefits:
- Enhanced Fraud Detection: NLP extends fraud detection beyond transaction monitoring by analyzing communications and behavior.
- Data Enrichment: NLP adds another layer of data to fraud detection efforts, helping to uncover hidden risks.
Benefits of AI for Risk Management and Fraud Detection
1. Increased Efficiency
AI can process and analyze data far more quickly than human analysts, allowing financial institutions to make faster decisions and respond to risks in real time. This increased efficiency leads to faster fraud detection, quicker risk assessments, and reduced manual workloads.
2. Cost Savings
By automating risk management and fraud detection processes, AI reduces the need for manual intervention, saving financial institutions significant operational costs. Additionally, AI-driven solutions can lower the costs associated with fraud losses and regulatory fines.
3. Improved Accuracy
AI systems continuously learn and adapt from data, improving their accuracy in identifying risks and detecting fraud over time. This results in more accurate predictions, fewer false positives, and more effective prevention of fraudulent activities.
4. Scalability
As financial institutions grow and handle more transactions, AI-powered solutions can easily scale to accommodate increasing data volumes. This scalability ensures that institutions can maintain high levels of security and risk management without significant increases in resources.
Challenges of Implementing AI in Financial Services
While AI offers tremendous benefits for risk management and fraud detection, it is not without challenges.
1. Data Quality and Availability
AI relies on high-quality data to make accurate predictions. If data is incomplete, inconsistent, or biased, the AI models may produce inaccurate results. Ensuring that the necessary data is available and properly cleaned is essential for successful AI implementation.
2. Regulatory Compliance
Financial institutions must navigate complex regulatory environments when adopting AI. AI solutions must comply with data privacy regulations, anti-money laundering (AML) rules, and other financial regulations, which can be challenging to implement.
3. Talent and Expertise
Building and maintaining AI solutions requires specialized knowledge in data science, machine learning, and AI development. Many financial institutions face a shortage of skilled professionals who can manage AI projects.
Best Practices for Implementing AI in Financial Services
- Invest in High-Quality Data: Ensure that data is accurate, complete, and well-structured to maximize the effectiveness of AI models.
- Collaborate with Experts: Work with AI and machine learning experts to develop and implement robust AI solutions tailored to your institution’s needs.
- Ensure Regulatory Compliance: Stay up to date with the latest regulations to ensure that AI-driven processes meet legal and compliance requirements.
- Start Small and Scale Gradually: Begin with small AI projects that address specific risk or fraud detection challenges and scale them as you gain more experience and confidence in the technology.
Conclusion
AI is reshaping risk management and fraud detection in the financial services industry, providing institutions with powerful tools to mitigate risks and stay ahead of evolving threats. By automating processes, improving accuracy, and offering real-time insights, AI can help financial institutions operate more securely and efficiently.