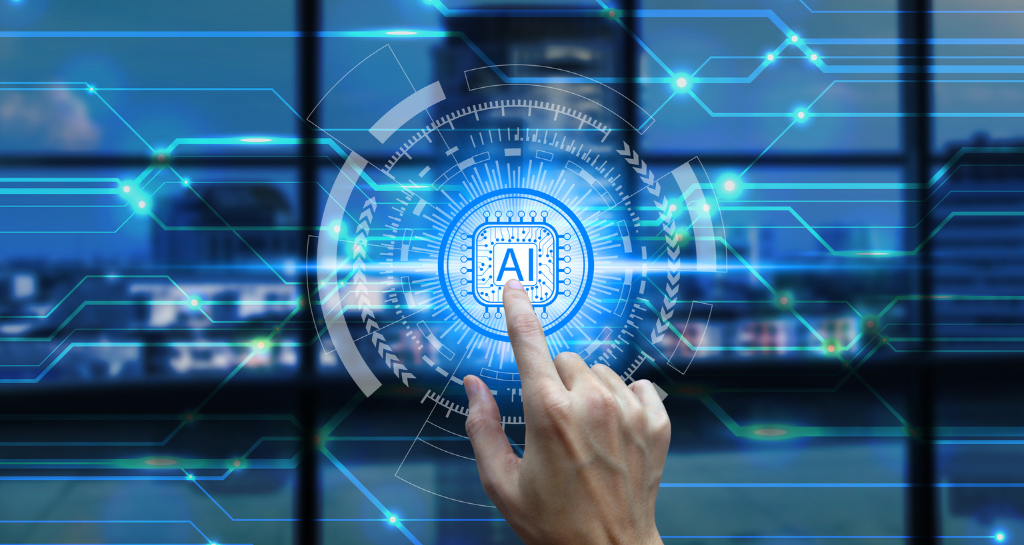
Deep Learning and AI: What Businesses Need to Know
Artificial Intelligence (AI) and deep learning are no longer just buzzwords — they are powerful technologies transforming industries across the globe. Businesses are leveraging AI and deep learning to automate tasks, gain deeper insights from data, and improve customer experiences. However, as these technologies rapidly evolve, many business leaders are left wondering: what exactly is deep learning, and how can it benefit their operations? In this blog, we will explore the fundamentals of deep learning and AI, the key differences between them, and the practical applications that businesses need to know to stay competitive.
Understanding AI and Deep Learning
1. What is Artificial Intelligence (AI)?
AI refers to the broad concept of machines being able to perform tasks that typically require human intelligence. This can include anything from recognizing speech and images to making decisions and translating languages. AI encompasses a variety of techniques, including machine learning, natural language processing (NLP), and robotics, to enable machines to mimic human cognitive functions.
Example:
- AI in Customer Support: AI-powered chatbots can handle customer queries 24/7, providing instant responses and resolving common issues.
2. What is Deep Learning?
Deep learning is a subset of machine learning, which in turn is a subset of AI. Deep learning uses neural networks modeled after the human brain to process large amounts of data and make decisions. It involves multiple layers of neurons, known as artificial neural networks, that "learn" from vast datasets to recognize patterns, make predictions, and even understand complex concepts without human intervention.
Example:
- Deep Learning in Image Recognition: Deep learning algorithms can analyze and classify millions of images to detect objects, faces, or anomalies in real time.
How Deep Learning and AI Differ
Although deep learning is a subset of AI, it is distinct in how it processes and learns from data. Here's a breakdown:
- AI: Refers to the general capability of machines to perform intelligent tasks, such as decision-making, speech recognition, and translating languages.
- Machine Learning: A subset of AI that enables systems to learn and improve from experience without being explicitly programmed.
- Deep Learning: A subset of machine learning that uses neural networks with multiple layers (hence "deep") to model complex patterns and representations.
Practical Applications of Deep Learning and AI for Businesses
Now that we have an understanding of AI and deep learning, let’s explore how these technologies are impacting business operations and how companies can take advantage of them.
1. Enhanced Customer Experience
AI and deep learning can be used to personalize customer interactions, offering tailored recommendations and services based on individual preferences and behaviors. By analyzing customer data, deep learning algorithms can predict future behaviors and offer personalized experiences in real time.
Example:
- Recommendation Engines: E-commerce platforms use deep learning to analyze browsing patterns and recommend products based on a customer’s previous interactions, increasing sales and engagement.
2. Automating Repetitive Tasks
AI can automate a wide range of repetitive and time-consuming tasks, freeing up employees to focus on higher-value work. From automated data entry to invoice processing, AI can handle mundane tasks faster and with fewer errors.
Example:
- Robotic Process Automation (RPA): Businesses can use AI-powered RPA to automate data entry, payroll processing, and other routine back-office functions, reducing manual errors and improving efficiency.
3. Predictive Maintenance
In industries such as manufacturing, AI and deep learning are being used to predict equipment failures before they occur. By analyzing sensor data in real time, deep learning models can detect patterns that indicate wear and tear, allowing businesses to perform maintenance proactively.
Example:
- Predictive Maintenance in Manufacturing: IoT sensors on machines can collect real-time data, which is then analyzed by AI models to predict when maintenance is needed, minimizing downtime and reducing repair costs.
4. Improved Decision-Making
AI and deep learning can analyze large datasets at a speed and accuracy far beyond human capability. Businesses can use these insights to make informed decisions in areas such as pricing, product development, and marketing strategies.
Example:
- Data-Driven Marketing: Deep learning algorithms can analyze customer data from multiple channels to identify trends, helping businesses tailor their marketing campaigns and allocate budgets more effectively.
5. Fraud Detection
In finance, deep learning algorithms are being used to detect fraud by analyzing transaction data and identifying unusual patterns in real time. This reduces the risk of fraud and improves overall financial security.
Example:
- Fraud Detection in Banking: AI-powered systems can monitor credit card transactions and alert financial institutions to suspicious activity, preventing fraudulent transactions and protecting customers.
6. Natural Language Processing (NLP)
NLP, a branch of AI, enables machines to understand, interpret, and generate human language. Businesses are using NLP to improve customer service, analyze sentiment, and enhance communication.
Example:
- Sentiment Analysis: AI-driven sentiment analysis tools can analyze customer reviews, social media posts, and feedback to gauge customer sentiment, helping businesses improve their products and services.
Challenges Businesses Face with AI and Deep Learning
Despite the enormous potential of AI and deep learning, businesses face several challenges in adopting these technologies.
1. Data Availability and Quality
Deep learning models require vast amounts of high-quality data to function effectively. For many businesses, acquiring and cleaning the necessary data can be a significant hurdle.
Solution:
- Invest in data infrastructure and ensure that your data is properly cleaned, labeled, and structured for use in AI models.
2. Talent Shortage
Implementing AI and deep learning solutions requires expertise in data science, machine learning, and software development. However, there is a global shortage of talent in these fields, making it difficult for businesses to find qualified professionals.
Solution:
- Consider partnering with AI consultants or outsourcing AI development to specialist firms.
3. Cost and Resources
AI and deep learning technologies often require significant computational resources, making them costly to implement, especially for smaller businesses.
Solution:
- Start with small-scale AI projects that can deliver quick wins, and leverage cloud-based AI platforms to reduce upfront infrastructure costs.
Best Practices for Businesses Implementing AI and Deep Learning
1. Identify Business Goals
Before implementing AI or deep learning, clearly define the business problems you aim to solve. Whether it's improving customer experiences, optimizing operations, or enhancing decision-making, having a clear focus will help guide your AI strategy.
2. Invest in Data Infrastructure
Ensure that your business has the necessary infrastructure to collect, store, and analyze data effectively. Without high-quality data, AI and deep learning models will not be able to perform optimally.
3. Start Small
Begin with small-scale AI projects that have clear, measurable outcomes. This allows your business to build expertise and demonstrate the value of AI before committing to more significant investments.
4. Continuously Monitor and Improve Models
AI models are not static — they need to be continuously monitored and updated to ensure they remain accurate and relevant. Regularly review your models and retrain them as needed to account for changing data and business conditions.
Conclusion
AI and deep learning are transforming industries and reshaping how businesses operate, offering significant opportunities for automation, data analysis, and personalized customer experiences. However, to unlock the full potential of these technologies, businesses must be prepared to invest in the right infrastructure, talent, and data strategies. By starting small and focusing on specific use cases, businesses can gradually integrate AI and deep learning into their operations, driving growth and staying competitive in an increasingly digital world.