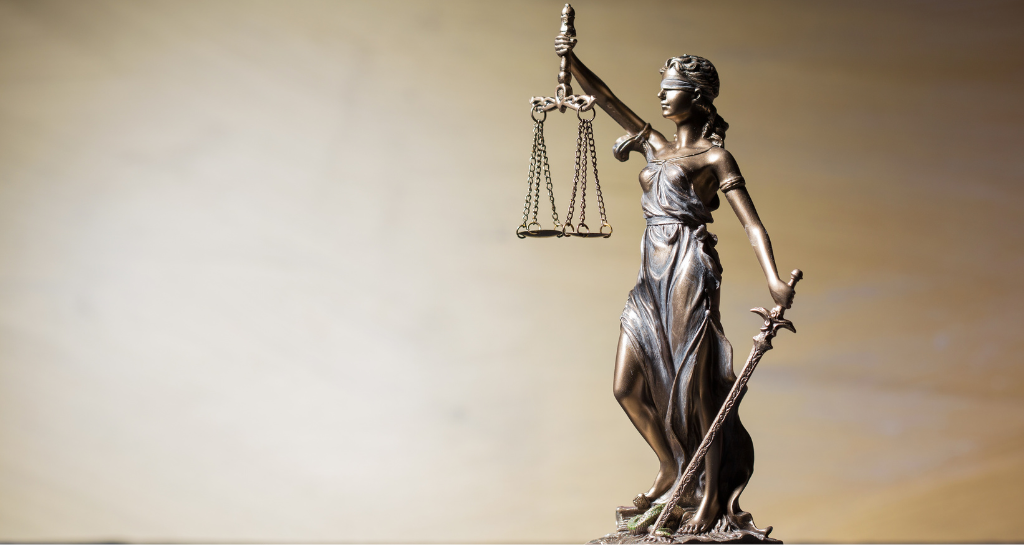
Ethical Considerations in AI: What Businesses Should Consider
As Artificial Intelligence (AI) becomes more ingrained in business operations, from decision-making to customer service, ethical considerations are taking center stage. AI’s ability to process large amounts of data, automate tasks, and make predictions brings tremendous value to businesses, but it also raises critical ethical questions. From data privacy and bias to transparency and accountability, companies adopting AI must navigate these challenges carefully. As a technology consultant who helps businesses integrate AI, I’ve seen how thoughtful approaches to AI ethics can lead to more sustainable and responsible innovation. In this blog, we’ll explore the key ethical considerations that businesses should keep in mind when implementing AI technologies.
Why Ethics Matter in AI
AI, by its very nature, can have profound impacts on individuals, communities, and society at large. When designed and deployed ethically, AI can offer tremendous benefits, such as improving efficiency, reducing human error, and enhancing customer experiences. However, unethical AI practices can lead to unintended consequences like privacy violations, discrimination, and loss of trust.
For businesses, ethical AI is not just a moral imperative—it’s a practical one. Companies that fail to prioritize ethical considerations risk damaging their reputation, facing legal penalties, and losing customer trust. By approaching AI with an ethical mindset, businesses can build solutions that are fair, transparent, and respectful of human rights.
Key Ethical Considerations in AI for Businesses
1. Bias and Fairness in AI Algorithms
AI systems learn from the data they are trained on, and if that data reflects existing societal biases, the AI can perpetuate or even amplify those biases. This can lead to unfair treatment of certain groups, especially in areas like hiring, lending, and law enforcement. Ensuring fairness in AI systems is one of the most significant ethical challenges businesses face.
Example:
- AI in Hiring: An AI algorithm used to screen job applicants may favor certain demographic groups over others if the training data is biased toward past hires who come from a particular gender, race, or background.
What Businesses Should Do:
- Diverse Data: Ensure that the data used to train AI models is diverse, representative, and free from bias. Regularly audit the data for any skewed patterns.
- Algorithm Auditing: Regularly audit AI algorithms to check for any biased outputs or discriminatory outcomes. Ensure that fairness metrics are built into the development process.
- Inclusive Teams: Create diverse teams to develop, test, and monitor AI systems to help identify and mitigate potential biases.
2. Data Privacy and Security
AI systems require vast amounts of data to function effectively. However, the collection, storage, and use of personal data raise significant privacy and security concerns. Businesses must ensure that they handle customer data ethically and comply with data protection laws such as the General Data Protection Regulation (GDPR) and the California Consumer Privacy Act (CCPA).
Example:
- AI in Healthcare: AI-driven healthcare platforms collect sensitive personal data from patients, such as medical records, genetic information, and health metrics. If this data is not adequately protected, it could be exposed in a data breach or used without consent.
What Businesses Should Do:
- Informed Consent: Ensure that customers are informed about how their data will be used, and obtain explicit consent before collecting personal data.
- Data Minimization: Collect only the data necessary for the AI system to function, and avoid gathering excessive information that isn’t directly relevant.
- Security Measures: Implement robust data protection protocols, including encryption and access controls, to safeguard sensitive information.
3. Transparency and Explainability
AI systems can be complex, and their decision-making processes are often opaque. This “black box” nature of AI makes it difficult for businesses and users to understand how decisions are made. Lack of transparency can lead to a loss of trust, especially in high-stakes industries such as healthcare, finance, and law enforcement.
Example:
- AI in Lending: An AI system might deny a loan application based on factors that the applicant cannot see or understand, leading to frustration and lack of trust in the institution.
What Businesses Should Do:
- Explainable AI: Invest in explainable AI (XAI) technologies that allow stakeholders to understand how AI models make decisions. Transparency fosters trust and accountability.
- Clear Communication: Be transparent with customers about how AI is used in decision-making processes, and provide explanations for decisions that impact them.
- Human Oversight: Ensure that AI systems used in critical decision-making processes are overseen by humans who can interpret and question AI outputs when necessary.
4. Accountability in AI Decision-Making
AI systems, especially those deployed in autonomous roles, can have real-world consequences, from making hiring decisions to determining loan eligibility or even diagnosing medical conditions. When things go wrong, who is accountable? Businesses must establish clear guidelines for accountability in AI decision-making.
Example:
- Self-Driving Cars: If an autonomous vehicle controlled by AI causes an accident, it raises questions about who is responsible—the car manufacturer, the software developer, or the car owner?
What Businesses Should Do:
- Accountability Frameworks: Develop clear accountability frameworks that outline who is responsible for AI outcomes, particularly in high-stakes applications.
- Human-in-the-Loop Systems: In critical decision-making areas, ensure that humans remain in control and can intervene if AI systems make questionable decisions.
- Ethical AI Governance: Establish internal committees or external advisory boards to oversee AI ethics, ensuring that the technology aligns with organizational values and ethical standards.
5. Impact on Employment and the Workforce
AI-driven automation has the potential to displace jobs, particularly in industries such as manufacturing, logistics, and customer service. While AI can boost productivity and efficiency, it’s important for businesses to consider the impact of automation on workers and take steps to mitigate job loss.
Example:
- AI in Customer Service: AI chatbots can handle customer queries, reducing the need for human customer service representatives. While this improves efficiency, it may also lead to layoffs.
What Businesses Should Do:
- Reskilling Programs: Invest in upskilling and reskilling programs to help employees transition to new roles as AI automates repetitive tasks.
- Job Creation: Use AI to augment human capabilities rather than replace them. Many AI systems require human oversight, and new job opportunities can be created in AI development, monitoring, and maintenance.
- Ethical Automation Strategies: Adopt responsible automation strategies that consider the long-term impact on the workforce and provide support for employees affected by AI-driven changes.
6. Environmental Impact of AI
Training and running AI models, especially deep learning algorithms, can be computationally intensive, requiring significant energy consumption. As AI adoption grows, businesses must consider the environmental footprint of their AI systems.
Example:
- AI in Data Centers: Training large-scale AI models in data centers can consume enormous amounts of energy, contributing to carbon emissions and environmental degradation.
What Businesses Should Do:
- Energy Efficiency: Prioritize energy-efficient AI models and optimize the infrastructure used for training and deploying AI systems.
- Sustainable AI Practices: Explore renewable energy sources and green data centers to reduce the environmental impact of AI operations.
- Environmental Accountability: Be transparent with stakeholders about the environmental impact of AI, and set goals for reducing energy consumption and carbon emissions.
Best Practices for Ethical AI Implementation
1. Establish Ethical AI Guidelines
Develop clear, organization-wide ethical guidelines for AI development and deployment. These guidelines should address issues like bias, transparency, accountability, and privacy, and they should be regularly updated as technology evolves.
2. Conduct Ethical Audits
Regularly audit AI systems to ensure they comply with ethical standards. This includes evaluating data sources, testing for bias, and reviewing decision-making processes.
3. Foster a Culture of Ethical Responsibility
Build a company culture that prioritizes ethical AI. Train employees on ethical considerations, encourage open discussions about the ethical implications of AI projects, and make ethics a core part of the development process.
4. Collaborate with External Experts
Consider working with external ethical AI experts, advisory boards, or third-party auditors to provide an unbiased perspective on your AI systems and ensure compliance with ethical standards.
Conclusion
As businesses continue to adopt AI technologies, addressing the ethical implications of AI becomes crucial for long-term success and societal impact. From mitigating bias to ensuring transparency and accountability, ethical AI is not just about avoiding harm but also about building trust and fostering responsible innovation.